Services
SYGNOMICS Precision Oncology
We have developed an innovative paradigm to use a patient-specific disease network delineated by SYGNAL to contextualize a universe of knowledge of cancer biology from laboratory and clinical studies and enable personalized actionable insights for an individual patient.

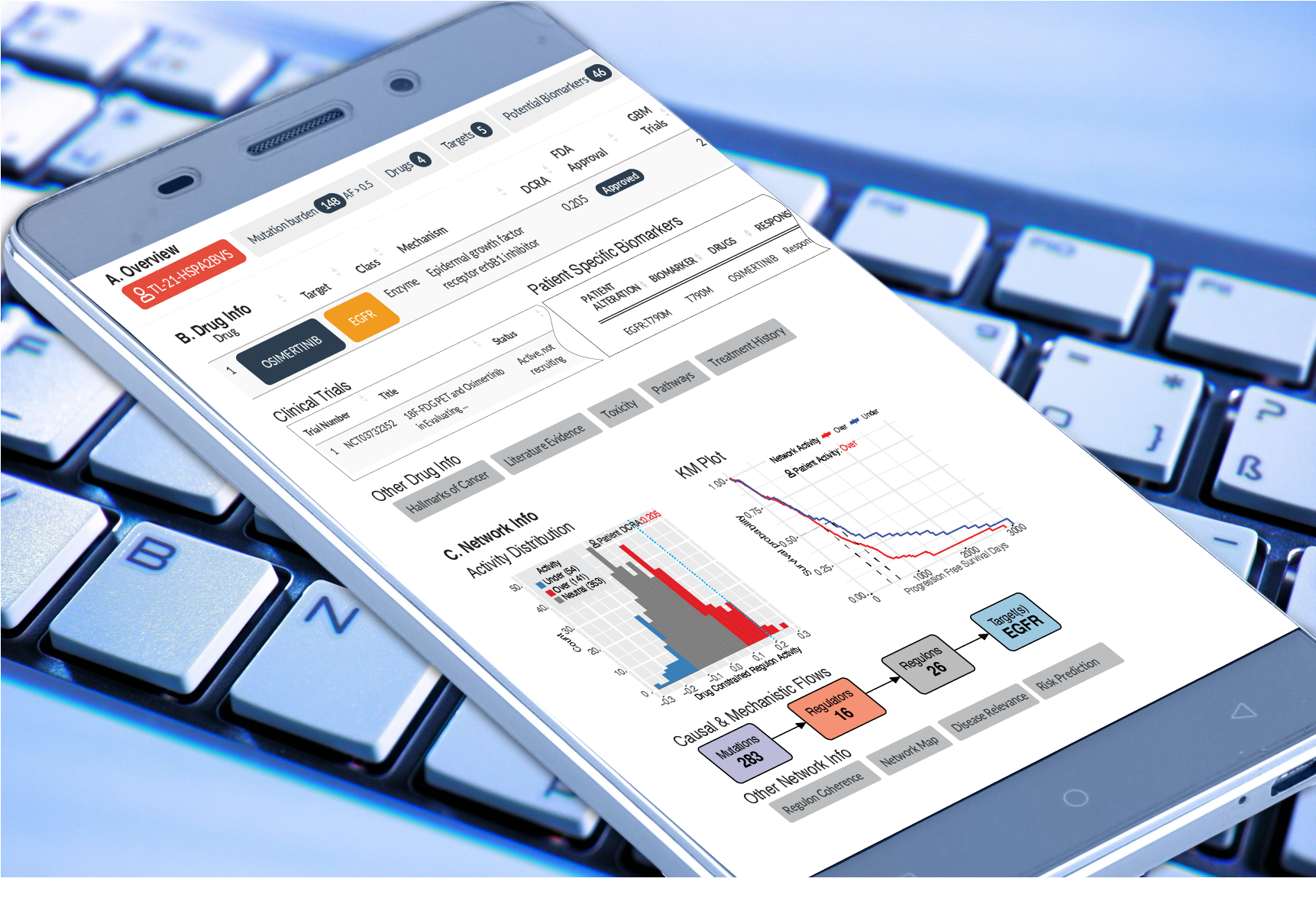
Sygnomics CDSS
Clinical Decision Support System (CDSS)
The clinical decision support system (CDSS) provides functionality for oncologists to ‘browse’ internally vetted and shortlisted systems biology-based insights for an individual patient, contextualized with evidence from diverse sources, including outcomes of similar patients within large cohorts, clinical trials, and literature insights. This allows the oncologists to rapidly synthesize an overwhelming body of multi-omic and literature-based information to make treatment decisions in real-time.
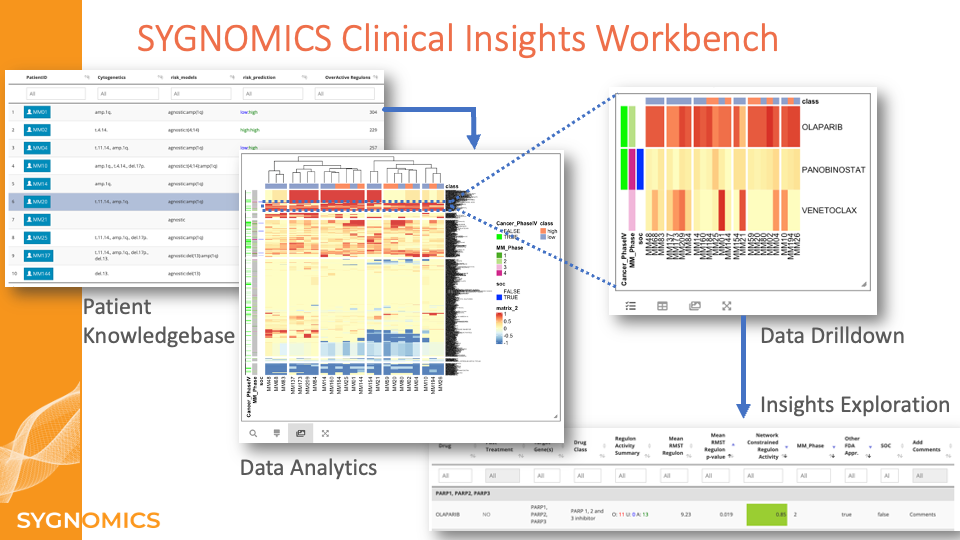
Sygnomics CIW
Clinical Insights Workbench (CIW)
The Clinical Insights Workbench (CIW) supports diverse drug discovery efforts, including novel target identification, biomarker-enriched trials, model-based repurposing of existing FDA-approved drugs, and companion diagnostics to match therapies to patients of specific characteristics.
Unprecedented advancements in technologies to probe molecular profiles of individual patients are creating a massive amount of data. This data revolution is empowering physicians to help individual patients through data-driven risk prediction and therapeutic decisions. As the amount of data increases exponentially, it is presenting enormous opportunities and challenges to extract meaningful insights that can aid us to grasp disease mechanisms, predict therapy responsiveness and identify novel drug targets. However, researchers, biopharma companies, and clinicians are struggling to effectively use these massive amounts of data to distill unique insights due to the vast amount of that is unstructured, noisy, and often disconnected from other relevant information. Driven by the need to translate our novel systems biology approach to personalized and intuitive patient risk and therapy recommendation reports, we have developed the Sygnomics Clinical Insights Workbench (CIW). Built on top of the SYGNAL machine learning algorithm, CIW leverages propriety Sygnomics Knowledgebase that includes multi-dimensional patient data, risk prediction, therapy recommendation, and other supporting information from disparate data sources. Clinical insights workbench facilitates congregating data from multiple sources such as clinical trials, drug toxicity, etc., and provides an intuitive interface to visualize trends and insights that are distilled from our comprehensive molecular profiling data with novel machine learning algorithms. CIW allows researchers to use automated operational tasks such as complex comparisons or clustering while also making it possible to manually control each parameter.
Clinical researchers can explore a global view of data and use integrated computational tools to query and interpret complex patterns to discover novel insights such as the networks that are activated only in a subset of patients and the potential mechanisms underlying these networks. In addition, Pharma companies can accurately classify a subset of patients who are highly likely to respond to given therapies or they can re-purpose drugs to match patients’ network activities.
In addition, SCIW can facilitate sharing and collaboration of data, analyses, and other insights across different researchers/companies.
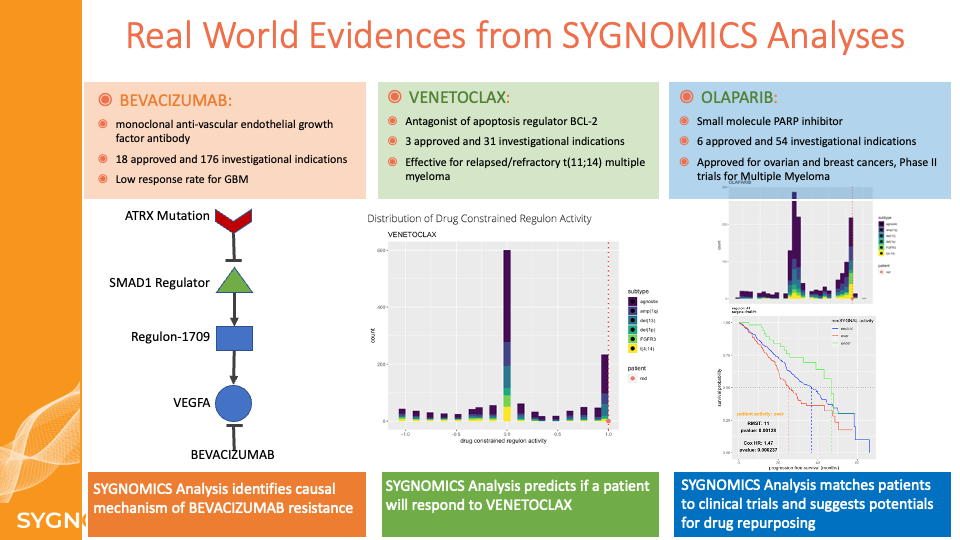
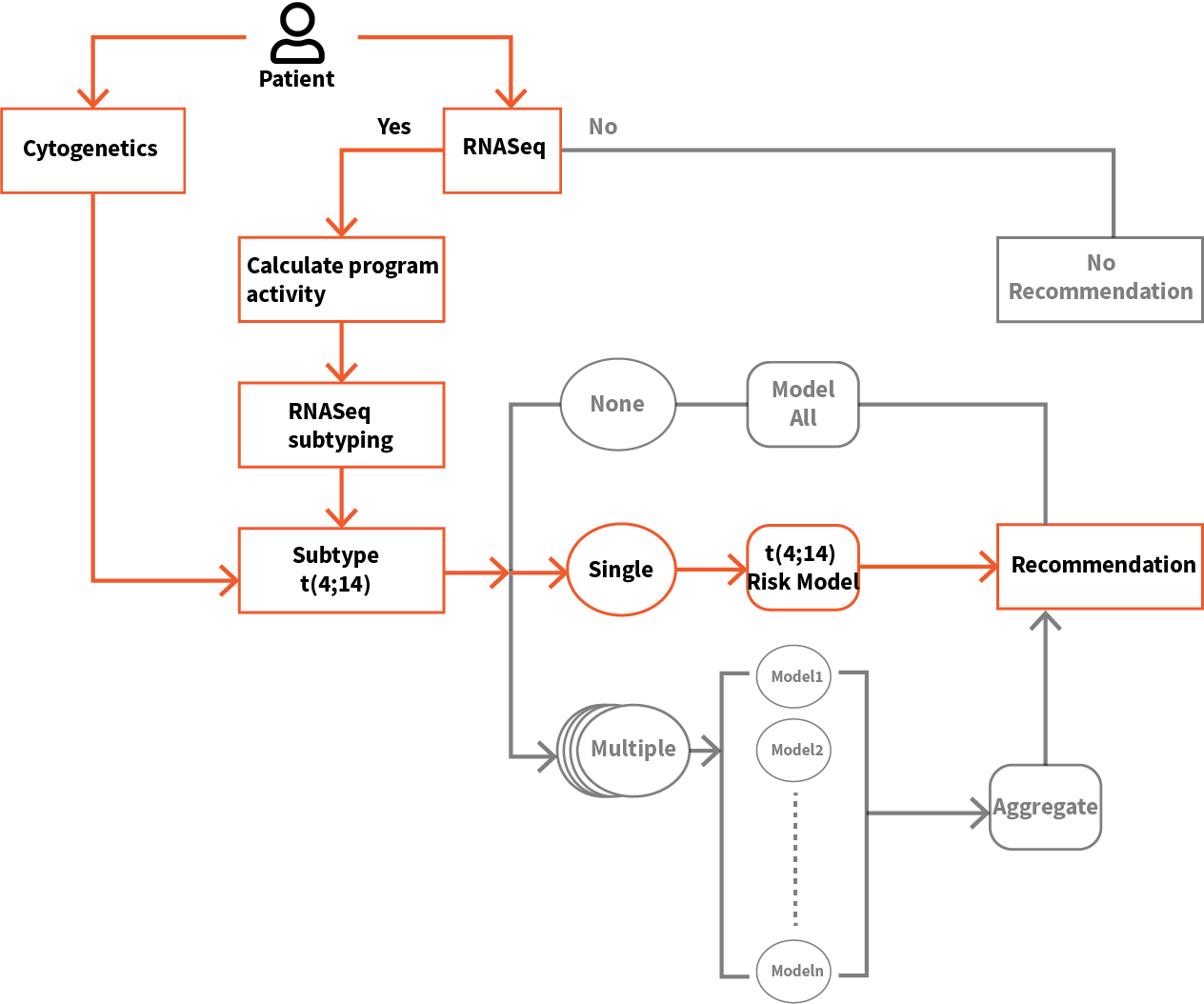
Risk Prediction
Poor risk assessment can lead to the selection of a suboptimal treatment plan, significantly lowering a patient’s quality of life and incurring higher healthcare costs. We have leveraged SYGNAL-enabled systems level understanding of an individual patient’s disease to develop a prognostic tool for dynamic risk assessment. This prognostic test to assess the severity of cancer progression at varied stages can have transformational implications on improving outcomes by enabling dynamic calibration of personalized treatment plans based on the unique disease trajectory of each patient.
Many studies have used gene expression profiling to better understand subtypes and stages of cancer progression. For example for Multiple Myeloma, two multigene biomarker panels, SKY92 (also known as EMC-92) and GEP70 (also known as UAMS-70), that use expression patterns of 92 and 70 genes, respectively, were commercialized into clinical tests to predict the risk of disease progression. However, since SKY92 and GEP70 models were trained on transcriptome profiles from MM samples obtained at the time of primary diagnosis, they are not as useful for risk assessment in the later stages of the disease.
We applied elastic net regression, a type of machine learning (ML), to identify specific programs within the SYGNAL network, whose activity profiles accurately predicts risk of disease progression in each individual across a patient cohort. By training the ML algorithm on subsets of patients, we have also developed models that provide finer-grade risk stratification within cytogenetic subtypes of cancer. We have combined multiple models into a SYGNAL risk prediction framework that was tested on three independent Multiple Myeloma cohorts (two microarray datasets from cohorts of newly diagnosed patients and one RNASeq dataset from a prospective double-blind study on a cohort of patients sampled at varied stages of the disease). Through these independent cohort studies, we demonstrate that risk prediction with mmSYGNAL significantly outperforms cytogenetics and multi-gene biomarker panels (SKY92 and GEP70). Importantly, we demonstrate that mmSYGNAL accurately predicts the risk of progression at any stage of the disease –at primary diagnosis, pre- or post-transplant, and even after multiple relapses. Finally, we discuss how the causal and mechanistic underpinnings of genetic programs used for risk prediction with mmSYGNAL also provide actionable insight into the selection of appropriate therapies for each patient.
Publication: Murie et al., Prognostic test for longitudinal monitoring of disease progression risk in multiple myeloma. (in preparation)
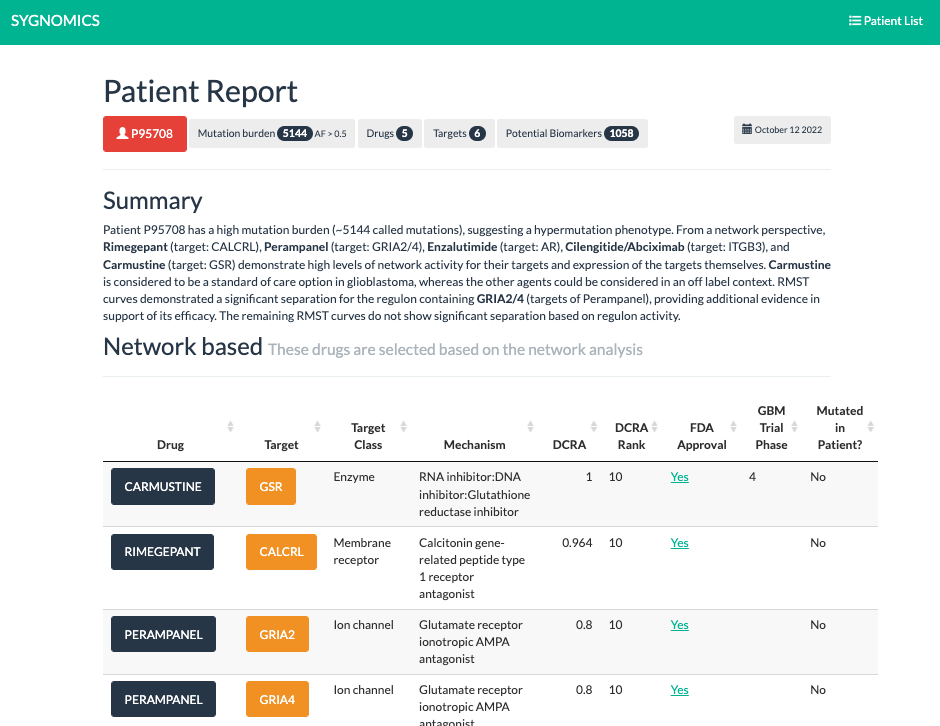
Therapy Matching
We have demonstrated that the activity status of programs inferred by SYGNAL analysis of the transcriptome profile of a patient’s tumor cells captures both the consequences of mutations and the influence of the tumor microenvironment on disease progression and therapy responsiveness. By leveraging this breakthrough we have developed a strategy to prioritize therapies that are likely to be effective for an individual patient.
A significant number of drugs have been approved as individual, double, triple and even quadruple combination therapies for the treatment of different cancers. While there are many choices of therapies, a rational strategy for selecting appropriate therapies based on the unique disease characteristics of a given patient is lacking. The current strategy is empirical and builds on rudimentary knowledge, such as the stage of diagnosis, cytogenetic features, and the treatment history of a patient. However, cancer is a heterogeneous disease with multiple mutations acting in complex combinations and interactions with other cells (e.g., osteoblasts) in the unique microenvironment of a patient’s tumor, which can significantly alter the drug sensitivity profiles of cancer cells. This is why mutations by themselves are poor predictors of disease phenotypes and therapy responses in each patient. As a result, some patients may never receive the correct treatment, and there is concern that administering the wrong therapy may do more harm than good. Even when therapies do work, in most cases, it is not clear why some patients responded and others did not. Hence, there is an urgent and unmet need for a strategy to tailor therapies to the unique characteristics of a patient’s disease.
We have demonstrated that the activity status of programs inferred by SYGNAL from the transcriptome profile of myeloma cells from a patient’s biopsy captures both the consequences of mutations and the influence of the tumor microenvironment on disease progression and therapy responsiveness. For instance, program activities within the mmSYGNAL model explained why some t(4;14) patients respond to SOC therapies, but other t(4;14) patients do not and are at significantly higher risk of progressing. mmSYGNAL also predicted FDA-approved drugs that are likely to be effective because they target overactive programs contributing to the increased risk of disease progression in these high and extreme-risk t(4;14) patients. Notably, some drugs such as PARP1 inhibitors identified by this approach have shown enduring responses in some RRMM patients, when administered with dexamethasone and bortezomib.